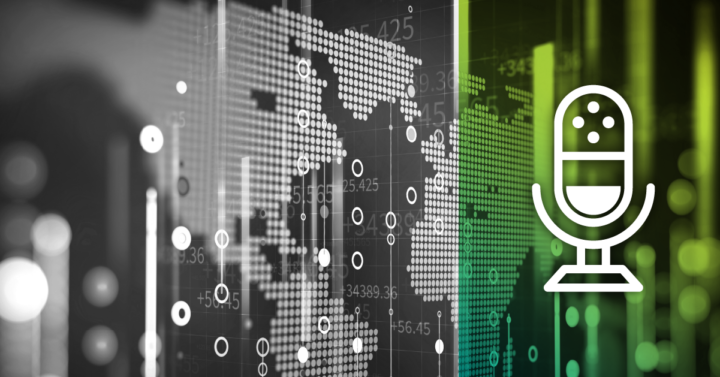
Big data and risk classification: Understanding the actuarial and social issues is the CIA’s latest policy statement. In this episode of the podcast, Matt Buchalter, FCIA and Chris Cooney, FCIA speak about the need for this statement, the benefits that increased data collection can have on insurance ratemaking and society, how policyholder privacy is paramount, and how data collection is expected to evolve.
NO TIME TO LISTEN? READ THE TRANSCRIPT.
Fievoli: Welcome to Seeing Beyond Risk, a podcast series from the Canadian Institute of Actuaries. I’m Chris Fievoli, Actuary, Communications and Public Affairs at the CIA.
The CIA has released a policy statement entitled Big data and risk classification: Understanding the actuarial and social issues. We encourage everyone to read this document, which is on the CIA website. And to provide some background on the discussions that led to the statement, we are joined by two members of the task force responsible for its creation, CIA members Matt Buchalter and Chris Cooney.
Thanks very much to both of you for joining us today.
Buchalter: Thanks, Chris. Great to be here.
Cooney: Likewise, thanks for having me.
Fievoli: So, let’s start off by maybe just recapping for us, “what’s the state of big data?” particularly in the P&C world, where you both practice. What are some examples of data that are being collected now that we just weren’t getting, say, 15 to 20 years ago?
Buchalter: I think the quintessential example of big data in the P&C world would be vehicle telematics devices, and that’s something that just started to come to prominence in Canada about 10 years ago.
And the way it works is it’s either a phone app or a piece of hardware that’s connected directly to the vehicle, and it can measure all kinds of things; and the benefits of a vehicle telematics device really come into two categories.
First, you get you a better measure of exposure, so, obviously, the more driving a given vehicle does in a given year the more opportunities it would have that to have a claim. And prior to telematics devices, the total mileage per year was something that had to be estimated by the customer, and there are a couple of problems with that.
Number one, no one really keeps track of how many kilometres they drive in a year, and even if they did, there’s not really a lot of ways to verify that information from the insurance point of view. So, you have the device built thoroughly into the vehicle, or through a phone app that can measure, very accurately, how many kilometres a year this vehicle is being driven.
In addition to that, the vehicle telematics device is providing a measure of risk – and by risk – in this case I mean driving behaviours, whether it’s hard acceleration, hard braking, hard cornering. And some of the devices have different ways that they can measure driver fatigue. All these things allow the insurance company to have a much more detailed assessment of the risk of any given drive or driving any given vehicle than what would have been possible prior to the advent of telematics devices.
Cooney: And although I’m a property and casualty actuary, I’m also familiar with the fact that there are some Fitbit-type health-information applications. For example, I know that Manulife has the vitality program, where they offer certain incentives and rewards for their customers, really to encourage healthy lifestyles.
The one other thing I would note just in the realm of big data is that historically, and I’ve been around the industry a long time, we would have conducted a statistical analysis. We would generally have used aggregated statistics and you can look at, say, some of our industry data sources where they didn’t really have all the granularity and they didn’t have some of the intersections between, let’s say, driving age and the number of years licensed; you would get them sort of aggregated independently.
But today we tend to use much wider data sets, with a lot more supplemental information. It’s more granular, it’s at a risk level, and that’s another change that I think is really transforming the way analytics are performed in our industry.
Fievoli: I guess one question is why do we need more data? I mean, why could insurers not just keep using the data they’ve traditionally collected? Why do we have this need for more information?
Cooney: I think, in essence, there’s a lot of innovation and search for competitive advantage as a natural factor that takes us there. We also see that society is evolving around us, and I think the emergence of the smartphone and even the ability to capture telematics information is new and, of course, we’re going to see how we can use these new technologies to give us an advantage.
In the life space, I think DNA testing is widespread and many of us will have obtained information that connects us to an assessment of future health risks in addition to just the typical origins-type analysis. And again, from a data asymmetry perspective, where potential applicant knows more about their specific risks than an insurer, that’s always been a risk that’s been evaluated at the underwriting stage, and today this risk is even more elevated given the preponderance of some of these new insights.
Buchalter: I think big data is a fairly new concept, but it’s just a natural evolution of predictive analytics and risk classification that’s been going on at least in the P&C industry for 30+ years.
I agree with Chris that we operate in a highly competitive market. And this is a good thing for consumers because they can search for a carrier whose coverage and pricing is best for them, but it also leads to a bit of a data and analytics “arms race” because anti-selection is a problem that is real and that is prominent, and whoever in the industry has the most accurate, most sophisticated risk classification and pricing will be, I guess you could say, on the winning end of that adverse selection game.
And nobody wants to be on the losing end because that’s a big problem for any company financially. So, every insurance company is on a constant search for new data, new analytical techniques, in order to predict any individual’s risk as accurately as possible.
Cooney: That’s a great point, Matt. And also, the cost of data storage and the speed of computing power, and so on, it’s all been on an upward trajectory, of course, and with new methodologies and open source, you can go and get the latest and greatest statistical methodologies for free, effectively.
I think all of that is really kind of playing into, as Matthew called it, the arms race, as accelerating the arms race.
Fievoli: I want to talk a bit more about pricing in a couple of minutes. But first I want to do a little detour and talk a bit about data privacy, because that’s obviously an issue that comes up when we talk about big data. How is the industry managing policyholder expectations in this particular area?
Cooney: As someone who works in a large Canadian organization, I can reflect that internal policies and practices focus a great deal on customer privacy protection.
For example, we have internal guidelines that require submission of privacy assessments before we conduct research into a particular data and outcome relationship, all of our staff goes through training on a regular basis that talks about the importance of privacy and maintaining trust with consumers.
We take particular care with what we define as “PII” in the industry, or personally identifiable information, because we understand that the risk this poses to our customers is the greatest in that case, and of course, information security is another critical element because we can only protect the customer’s privately shared information when it’s within our ecosystem.
And, of course, if there’s a bridge to the external world, there’s a great deal of risk for our customers when their data is exposed.
Buchalter: I would just add that privacy and ethical data-collection practices are by no means issues that are unique to the insurance industry.
There are all kinds of strict laws and regulations about collection and use of personal information. There’s actually something that just came out recently, called the Digital Charter Implementation Act, which even further outlines responsibilities with regard to collection and use of personal information.
And these things apply to all industries, including insurance, and one of the basic legal principles of insurance is that it’s a transaction requiring the utmost good faith between the policyholder and the insurer. In my mind, that principle of utmost good faith extends to responsible data collection and usage. I believe the insurance industry holds itself to the highest possible standards with regard to ethical data collection, ethical data use, proper data storage and disposal of personal information.
Cooney: And I’ll just connect a little bit more to that term “usage” that you used, Matthew. Just in the sense that we very often look very closely at what the consent statement says. We will use the information for and, again, consent is a critical part of the privacy discussion, because if you consented for use for a particular purpose, and then the insurance company goes and turns around and does that in a different kind of use exercise, that’s a breach of trust.
As part of these privacy assessments that I was mentioning, that’s a critical element of the review that takes place before we go and use it. So, for example, UBI, or Usage-Based Insurance Information, gets shared, we need to make sure that when our analysis is conducted, it’s purely in the role of lost-cost analysis and isn’t going into other domains that were disclosed when the customer signed up for that particular program.
Fievoli: Let’s get back to the pricing issues. In particular, I’m curious how pricing and ratemaking models have had to adapt themselves in order to accommodate this new level of data collection. So, I am hoping you could share some of the new techniques that you’ve seen being used in this space.
Buchalter: I think that the first big change is just the sheer volume of data. If you’re analyzing a book with, let’s say, 100,000 policies in it, prior to this big data age that would have been 100,000 records, 100,000 sets of risk characteristics, each of them attached to claims experience on that policy in that given period of time.
But now, if I go back to the example of vehicle telematics, your device might be taking a snapshot every 10 minutes, every five minutes, every minute, every 30 seconds. The number of records per policy per term, and the number of fields per record, are both growing exponentially. That brings into play all kinds of issues around data storage, around big data analytics, artificial intelligence, machine learning, all of these things that weren’t really needed before we started entering this world of big data.
We’ve always had big data in insurance, but when it’s dealing with 100,000 records versus 100 million records, some of the ways of processing that data becomes quite different. We’ve had to adapt to that in our ratemaking.
Cooney: And I had alluded earlier to the evolution of modelling methodologies, and I think that’s also a key area of focus. So, for example, XGBoost is a model that is well known in the statistical community for having great predictive power.
And I note that there are new methodologies and deep-learning techniques that enable even more access to new types of information sources; for example, image data such as satellite imagery. We might want to, for example, evaluate the roof size by looking at satellite imagery data. And that’s one less question that we can actually ask the consumer when they call us to ensure their policy, so it has a consumer benefit as much as it has an insurance benefit.
We’re able to reach into new information sources to help us create a better customer experience. The other thing I’ll allude to from a future-based perspective is we are obviously all concerned around increasing storm frequencies and climate risk, and similarly, I think that’s probably an area for revolution in the future: how climate risk and some of those longer-term trends might intersect with the viability of our insurance markets from a catastrophic risk potential.
Fievoli: Now I understand that the more that we add data for risk-classification purposes that can create some – shall we say – unexpected consequences, and I was hoping you could share a couple of examples, either real or hypothetical, that you may have to deal with as someone who’s trying to determine a rate structure for insurance.
Buchalter: I think when you look at unexpected consequences that often come from prevention of using certain risk-classification variables where you get a law or regulation that is usually well-intentioned but can have some adverse unintended consequences.
And there’s an example from much earlier in my career. When I was working in auto insurance pricing, one of the provinces decided that driver age was no longer an acceptable rating factor for auto insurance. What companies started doing is switching their rating models from one based on “driver age” to one based on “number of years” licensed, which is obviously closely related to age in that the majority of drivers would initially become licensed around age 16, 17, 18.
But that’s not true for every driver. You might get some drivers that first got their driver’s licence much later in life. Maybe due to a change in their family status, or maybe because they moved from the city out to the suburbs, where they needed to drive. Or maybe they were a newcomer to Canada and first got a Canadian driver’s licence later in life.
The unintended consequences of this regulation were that you would now have, let’s say, a 50-year-old driver with two years’ licensed because they’ve got their license later in life. And because the rating system had shifted from one based on age to one based on years licensed, you had this 50-year-old with two years licensed paying premiums for auto insurance that were in line with what an 18-year-old with two years licensed would pay.
And I would argue that there are some serious differences in terms of driving behaviours between an 18-year-old with two years’ licensed and a 50-year-old with two years’ licensed.
And I’m sure that this was not the intent of the regulation, but just like water will always flow to the lowest point, whenever there are regulatory constraints, insurance companies and pricing actuaries are always going to get creative to see how they can innovate different ways of rating while staying within the regulatory guidelines.
I think that’s an example of what happened in this case. I think the lesson from that is when you get overly prescriptive regulation around what data elements can and cannot be used, there is a high risk of unintended consequences where you, as the regulator or as the lawmaker, might expect one set of outcomes and get something that is quite a bit different.
Cooney: I recall that our [Risk classification] committee discussed the intersection between what I’ll call market economics and social fairness a great deal, and we noted that it requires a balancing act. Some of the commentary reflected that actuaries cannot ignore potential social issues.
We heard that in many of the review comments on our paper and we fulsomely agree: we have a responsibility to the public good. We note as well that there is an equally important challenge that exists on the economic side is that actuaries were defenders of the resilience of the system of risk transfer.
I don’t know that that’s a really well-known responsibility that actuaries possess, but that’s something that came back to us, is that, as Matthew alluded to, when you create this discontinuity in the marketplace, the economic forces – and I recall that facility association did not introduce either year’s licensed when they eliminated age, and they ended up having all the young drivers in the market. So, they’re very real forces that come to bear.
Another example that I would highlight is that much has been made of the higher cost of rates in Brampton for auto insurance, but there hasn’t actually been a lot of study as to the underlying factors.
So, for example, Brampton (Ontario) has a lot of six-lane roads capable of high vehicle speeds. It’s a community that’s built for commuting and I would know, because I’ve lived in close proximity to Brampton, that making a left turn across three lanes of oncoming traffic is much more difficult than it is when you’re making a left turn across one lane of traffic.
These are the types of facts that rarely enter the conversation, and I think the use of more granular analytics; for example, where the collisions are occurring; what is the nature of the severity of an accident. So, for example, do the six-lane roads have more severe collisions where there are higher speeds?
I think that’s one way to bring greater focus to the problem and actually to help address some of the underlying issues that are responsible for the higher cost. I like to say that our models point to the issues, what we do with them is a critical step that involves a lot of parties to help constructively address them.
Fievoli: Let’s wrap up by maybe taking a look forward. I’d like to ask you; how do you see data collection evolving over the next few years? Do you think we’ll start to collect more data, different types of data, or do we eventually reach a natural limit whereby we’ve done all that we can within our current pricing models?
Buchalter: There’s nothing static about big data and predictive analytics. It’s constantly evolving and improving, not only in insurance but everything from sports to movie recommendations to shopping. And I would say we are no exception to that. Who knows what the future will hold, but I would find it hard to believe that there will not continue to be improvements in data collection and analytics technology.
I think the only natural limit would be some imaginary future where everything is deterministic and predictable, and there is no randomness left in the world. I mean, that’s an interesting philosophical discussion to be had about whether such a world is physically possible, but I think for the near future, at least, it’s firmly in the domain of science fiction. So, I don’t see us running up against any natural limits any time soon in terms of predictive analytics, either in insurance, risk classification, or really in any other domain.
Cooney: The one thing I would call out, and I was on the actuarial conference [act22] presenting a segment around ESG [environmental, social, and governance] and focused really on the intersection of the social good and some of these issues.
And I think the one thing I would note is that, again, our market and social issues are bound to collide, and I think as we get to more granular levels of detail the potential for that is going to continue to increase. And one example I would raise again is territorial rating in auto, and should, for example, the auto industry moves to a postal-code-level rating, and I think that’s a place where, again, market forces will drive there.
But there are also social issues that we need to come to terms within response to that, and what I noted in my talk is that again we need to focus on this collaboratively. The regulator has to play a role. There’s also some bias and fairness protocols that occur within organizations that are also forcing us to confront this and to address it. The one thing we can’t do is ignore it, and as I noted in my talk, I think the actuarial community is a key player in helping to both understand where these issues are going to collide, and also helping raise awareness around how we can constructively address it.
We can’t always produce the solutions on our own because, as I mentioned, sometimes you need a regulator to help bridge a solution – maybe you need something like a pooling or sharing mechanism that can help to address some of the issues that are going to be merged – but the one thing we certainly can’t do is ignore it.
Fievoli: OK, lots of interesting issues. I’d like to thank both of you for coming on the podcast today to discuss them with us.
Cooney: Thanks, Chris.
Buchalter: Thanks, Chris.
Fievoli: Once again, the statement on Big data and Risk classification can be found on the CIA website. And we now have over 100 episodes in our podcast series going back over the past three years, so we encourage you to subscribe. And you can do so through whatever platform you use to get your podcast content. We’d also like to hear from you. If you have any suggestions or ideas for episodes, you can send them to podcasts@cia-ica.ca.
And we’re always looking for content to put on our Seeing Beyond Risk blog. If you have some ideas that you would like to share with us, you can reach us at SeeingBeyondRisk@cia-ica.ca.
Until next time. I’m Chris Fievoli. And thank you for tuning in to Seeing Beyond Risk.
This transcript has been edited for clarity.